9 Prediction
INCOMPLETE DRAFT
…
The essential questions for this chapter are:
- …
- …
- …
In this chapter we will
Orientation to the question(s) and dataset(s) to be explored …
wricle_df <- read_csv(file = "resources/10-prediction/data/derived/wricle_formal_curated.csv")
locness_df <- read_csv(file = "resources/10-prediction/data/derived/locness_curated.csv")
learners_df <- wricle_df %>%
filter(native_language == "Spanish") %>%
mutate(student = "Spanish") %>%
mutate(type = "Learner") %>%
select(essay_id = id, type, student, essay)
glimpse(learners_df)
#> Rows: 689
#> Columns: 4
#> $ essay_id <chr> "A1-1", "A1-2", "A10-1", "A10-2", "A101-1", "A101-2", "A101-3…
#> $ type <chr> "Learner", "Learner", "Learner", "Learner", "Learner", "Learn…
#> $ student <chr> "Spanish", "Spanish", "Spanish", "Spanish", "Spanish", "Spani…
#> $ essay <chr> "In our present society gay people is asking for the same rig…
natives_df <- locness_df %>%
unite(col = doc_id, c("file_id", "essay_id"), sep = "-") %>%
mutate(doc_id = str_replace(doc_id, "\\.txt", "")) %>%
mutate(type = "Native") %>%
select(essay_id = doc_id, type, student, essay)
glimpse(natives_df)
#> Rows: 411
#> Columns: 4
#> $ essay_id <chr> "alevels1-1", "alevels1-2", "alevels1-3", "alevels1-4", "alev…
#> $ type <chr> "Native", "Native", "Native", "Native", "Native", "Native", "…
#> $ student <chr> "British", "British", "British", "British", "British", "Briti…
#> $ essay <chr> "The basic dilema facing the UK's rail and road transport sys…
nativeness_df <- rbind(learners_df, natives_df) # combine
nativeness_df %>%
unnest_tokens(output = "word", input = "essay") %>%
count(word, type) %>%
group_by(type) %>%
summarize(total_words = sum(n))
#> # A tibble: 2 × 2
#> type total_words
#> <chr> <int>
#> 1 Learner 640535
#> 2 Native 324269
nativeness_corpus <- nativeness_df %>%
corpus(text_field = "essay")
nativeness_corpus_summary <- nativeness_corpus %>%
summary(n = ndoc(nativeness_corpus))
nativeness_corpus_summary %>%
slice_head(n = 10)
#> Corpus consisting of 1100 documents, showing 1100 documents:
#>
#> Text Types Tokens Sentences essay_id type student
#> text1 210 496 26 A1-1 Learner Spanish
#> text2 253 655 29 A1-2 Learner Spanish
#> text3 280 722 30 A10-1 Learner Spanish
#> text4 177 389 15 A10-2 Learner Spanish
#> text5 245 636 19 A101-1 Learner Spanish
#> text6 219 692 17 A101-2 Learner Spanish
#> text7 213 615 16 A101-3 Learner Spanish
#> text8 319 914 23 A101-4 Learner Spanish
#> text9 253 654 19 A102-1 Learner Spanish
#> text10 310 816 21 A102-2 Learner Spanish
nativeness_corpus$doc_id <- 1:ndoc(nativeness_corpus)
nativeness_corpus %>%
docvars() %>%
slice_head(n = 5)
#> essay_id type student doc_id
#> 1 A1-1 Learner Spanish 1
#> 2 A1-2 Learner Spanish 2
#> 3 A10-1 Learner Spanish 3
#> 4 A10-2 Learner Spanish 4
#> 5 A101-1 Learner Spanish 5
nativeness_tokens <- nativeness_corpus %>%
tokens(what = "word", remove_punct = TRUE, remove_numbers = TRUE, remove_symbols = TRUE)
nativeness_tokens %>%
tokens_group(groups = type) %>%
head()
#> Tokens consisting of 2 documents and 1 docvar.
#> Learner :
#> [1] "In" "our" "present" "society" "gay" "people" "is"
#> [8] "asking" "for" "the" "same" "rights"
#> [ ... and 636,407 more ]
#>
#> Native :
#> [1] "The" "basic" "dilema" "facing" "the" "UK's"
#> [7] "rail" "and" "road" "transport" "system" "is"
#> [ ... and 321,936 more ]
nativeness_dfm <- nativeness_tokens %>%
dfm()
nativeness_dfm %>%
head(n = 5)
#> Document-feature matrix of: 5 documents, 28,129 features (99.24% sparse) and 4 docvars.
#> features
#> docs in our present society gay people is asking for the
#> text1 12 5 4 5 5 10 12 1 3 23
#> text2 26 2 1 4 0 10 14 0 1 45
#> text3 12 4 0 4 9 16 12 0 0 12
#> text4 15 1 0 1 0 9 7 0 1 10
#> text5 15 1 1 2 0 7 17 0 6 40
#> [ reached max_nfeat ... 28,119 more features ]
nativeness_dfm %>%
dfm_group(groups = type) %>%
head(n = 5)
#> Document-feature matrix of: 2 documents, 28,129 features (33.74% sparse) and 1 docvar.
#> features
#> docs in our present society gay people is asking for the
#> Learner 15518 1279 322 1269 520 5980 12931 33 4993 36728
#> Native 6357 584 109 422 6 1569 6307 12 3144 21090
#> [ reached max_nfeat ... 28,119 more features ]
nativeness_dfm %>%
topfeatures(n = 25)
#> the of to and in that a is it this
#> 57818 32821 31155 24158 21875 20847 19981 19238 11259 10357
#> be are they not for as people have with or
#> 10096 10085 8438 8196 8137 7977 7549 7506 6007 5568
#> their i on by because
#> 5192 5128 4820 4579 4511
nativeness_dfm %>%
textstat_frequency(n = 5, groups = type)
#> feature frequency rank docfreq group
#> 1 the 36728 1 689 Learner
#> 2 of 22095 2 689 Learner
#> 3 to 20417 3 689 Learner
#> 4 that 15929 4 689 Learner
#> 5 and 15836 5 689 Learner
#> 6 the 21090 1 410 Native
#> 7 to 10738 2 410 Native
#> 8 of 10726 3 410 Native
#> 9 and 8322 4 409 Native
#> 10 a 6827 5 410 Native
nativeness_dfm %>%
dfm_tfidf() %>%
textstat_frequency(n = 5, groups = type, force = TRUE)
#> feature frequency rank docfreq group
#> 1 abortion 1108 1 69 Learner
#> 2 marijuana 901 2 46 Learner
#> 3 education 746 3 204 Learner
#> 4 children 735 4 317 Learner
#> 5 sex 658 5 133 Learner
#> 6 he 803 1 199 Native
#> 7 his 573 2 194 Native
#> 8 candide 522 3 27 Native
#> 9 quote 402 4 26 Native
#> 10 caligula 394 5 11 Native
set.seed(4321) # make reproducible
num_docs <-
nativeness_dfm %>%
ndoc()
train_size <-
(num_docs * .75) %>% # get size of sample
round() # round to nearest whole number
train_ids <- sample(x = 1:num_docs, # population
size = train_size, # size of sample
replace = FALSE) # without replacement
nativeness_dfm_train <- nativeness_dfm %>%
dfm_subset(doc_id %in% train_ids)
nativeness_dfm_test <- nativeness_dfm %>%
dfm_subset(!doc_id %in% train_ids)
nativeness_dfm %>%
docvars() %>%
janitor::tabyl(type)
#> type n percent
#> Learner 689 0.626
#> Native 411 0.374
nativeness_dfm_train %>%
docvars() %>%
janitor::tabyl(type)
#> type n percent
#> Learner 516 0.625
#> Native 309 0.375
nativeness_dfm_test %>%
docvars() %>%
janitor::tabyl(type)
#> type n percent
#> Learner 173 0.629
#> Native 102 0.371
nb1 <- textmodel_nb(x = nativeness_dfm_train, y = nativeness_dfm_train$type)
summary(nb1)
#>
#> Call:
#> textmodel_nb.dfm(x = nativeness_dfm_train, y = nativeness_dfm_train$type)
#>
#> Class Priors:
#> (showing first 2 elements)
#> Learner Native
#> 0.5 0.5
#>
#> Estimated Feature Scores:
#> in our present society gay people is asking
#> Learner 0.0230 0.00186 0.000476 0.00193 7.62e-04 0.00889 0.0193 5.76e-05
#> Native 0.0174 0.00163 0.000315 0.00114 2.22e-05 0.00460 0.0176 2.96e-05
#> for the same rights that heterosexual some of
#> Learner 0.00747 0.0544 0.001318 0.000909 0.0238 3.06e-04 0.00476 0.0328
#> Native 0.00886 0.0582 0.000563 0.000252 0.0140 1.11e-05 0.00157 0.0298
#> this are to be allowed get married and
#> Learner 0.01148 0.01114 0.0300 0.01023 0.000326 0.001054 3.67e-04 0.0233
#> Native 0.00796 0.00745 0.0296 0.00932 0.000278 0.000763 8.52e-05 0.0224
#> adopt children request has been accepted
#> Learner 2.64e-04 0.00268 1.39e-05 0.00414 0.00187 0.000137
#> Native 3.71e-05 0.00119 2.22e-05 0.00435 0.00213 0.000141
coef(nb1) %>% head()
#> Learner Native
#> in 0.023045 1.74e-02
#> our 0.001856 1.63e-03
#> present 0.000476 3.15e-04
#> society 0.001929 1.14e-03
#> gay 0.000762 2.22e-05
#> people 0.008893 4.60e-03
predict(nb1, type = "prob") %>% # get the predicted document scores
tail # preview predicted probability scores
#> Learner Native
#> text1092 2.37e-164 1
#> text1094 3.18e-28 1
#> text1095 8.53e-49 1
#> text1096 1.83e-37 1
#> text1097 7.45e-39 1
#> text1098 2.35e-55 1
nb1_predictions <-
predict(nb1, type = "prob") %>% # get the predicted document scores
as.data.frame() %>% # convert to data frame
mutate(document = rownames(.)) %>% # add the document names to the data frame
as_tibble() %>% # convert to tibble
pivot_longer(cols = c("Learner", "Native"), # convert from wide to long format
names_to = "prediction", # new column for ham/spam predictions
values_to = "probability") %>% # probablity scores for each
group_by(document) %>% # group parameter by document
slice_max(probability, n = 1) %>% # keep the document row with highest probablity
slice_head(n = 1) %>% # for predictions that were 50/50
ungroup() %>% # remove grouping parameter
mutate(doc_id = str_remove(document, "text") %>% as.numeric) %>% # clean up document column so it matches doc_id in
arrange(doc_id) # order by doc_id
nb1_predictions %>%
slice_head(n = 10) # preview
#> # A tibble: 10 × 4
#> document prediction probability doc_id
#> <chr> <chr> <dbl> <dbl>
#> 1 text1 Learner 1 1
#> 2 text3 Learner 1 3
#> 3 text4 Learner 1 4
#> 4 text5 Learner 1 5
#> 5 text6 Learner 1 6
#> 6 text8 Learner 1 8
#> 7 text9 Learner 1 9
#> 8 text11 Learner 1 11
#> 9 text12 Learner 1 12
#> 10 text13 Learner 1 13
nb1_predictions_actual <-
cbind(actual = nb1$y, nb1_predictions) %>% # column-bind actual classes
select(doc_id, document, actual, prediction, probability) # organize variables
nb1_predictions_actual %>%
slice_head(n = 5) # preview
#> doc_id document actual prediction probability
#> 1 1 text1 Learner Learner 1
#> 2 3 text3 Learner Learner 1
#> 3 4 text4 Learner Learner 1
#> 4 5 text5 Learner Learner 1
#> 5 6 text6 Learner Learner 1
tab_class <-
table(nb1_predictions_actual$actual, # actual class labels
nb1_predictions_actual$prediction) # predicted class labels
caret::confusionMatrix(tab_class, mode = "prec_recall") # model performance statistics
#> Confusion Matrix and Statistics
#>
#>
#> Learner Native
#> Learner 516 0
#> Native 10 299
#>
#> Accuracy : 0.988
#> 95% CI : (0.978, 0.994)
#> No Information Rate : 0.638
#> P-Value [Acc > NIR] : < 2e-16
#>
#> Kappa : 0.974
#>
#> Mcnemar's Test P-Value : 0.00443
#>
#> Precision : 1.000
#> Recall : 0.981
#> F1 : 0.990
#> Prevalence : 0.638
#> Detection Rate : 0.625
#> Detection Prevalence : 0.625
#> Balanced Accuracy : 0.990
#>
#> 'Positive' Class : Learner
#>
predicted_class <- predict(nb1, newdata = nativeness_dfm_test)
actual_class <- nativeness_dfm_test$type
tab_class <- table(actual_class, predicted_class) # cross-tabulate actual and predicted class labels
caret::confusionMatrix(tab_class, mode = "prec_recall") # model performance statistics
#> Confusion Matrix and Statistics
#>
#> predicted_class
#> actual_class Learner Native
#> Learner 172 1
#> Native 9 93
#>
#> Accuracy : 0.964
#> 95% CI : (0.934, 0.982)
#> No Information Rate : 0.658
#> P-Value [Acc > NIR] : <2e-16
#>
#> Kappa : 0.921
#>
#> Mcnemar's Test P-Value : 0.0269
#>
#> Precision : 0.994
#> Recall : 0.950
#> F1 : 0.972
#> Prevalence : 0.658
#> Detection Rate : 0.625
#> Detection Prevalence : 0.629
#> Balanced Accuracy : 0.970
#>
#> 'Positive' Class : Learner
#>
9.2 Model training
Aim to use the create an abstraction of the patterns in the dataset
Feature engineering
Model selection
Model evaluation
The In Figure () Let’s consider the results from a hypothetical model of text classification on the SMS dataset I introduced at in this subsection.
- accuracy (measure of overall correct predictions)
- precision (measure of the quality of the predictions)
- Percentage of predicted ‘ham’ messages that were correct
- recall (measure of the quantity of the predictions)
- Percentage of actual ‘ham’ messages that were correct
- F1-score (summarizes the balance between precision and recall)
Avoiding overfitting
9.4 Evaluation
Evaluation of results
Relationship between predicted and actual classes in a confusion matrix as seen in Figure 9.1.
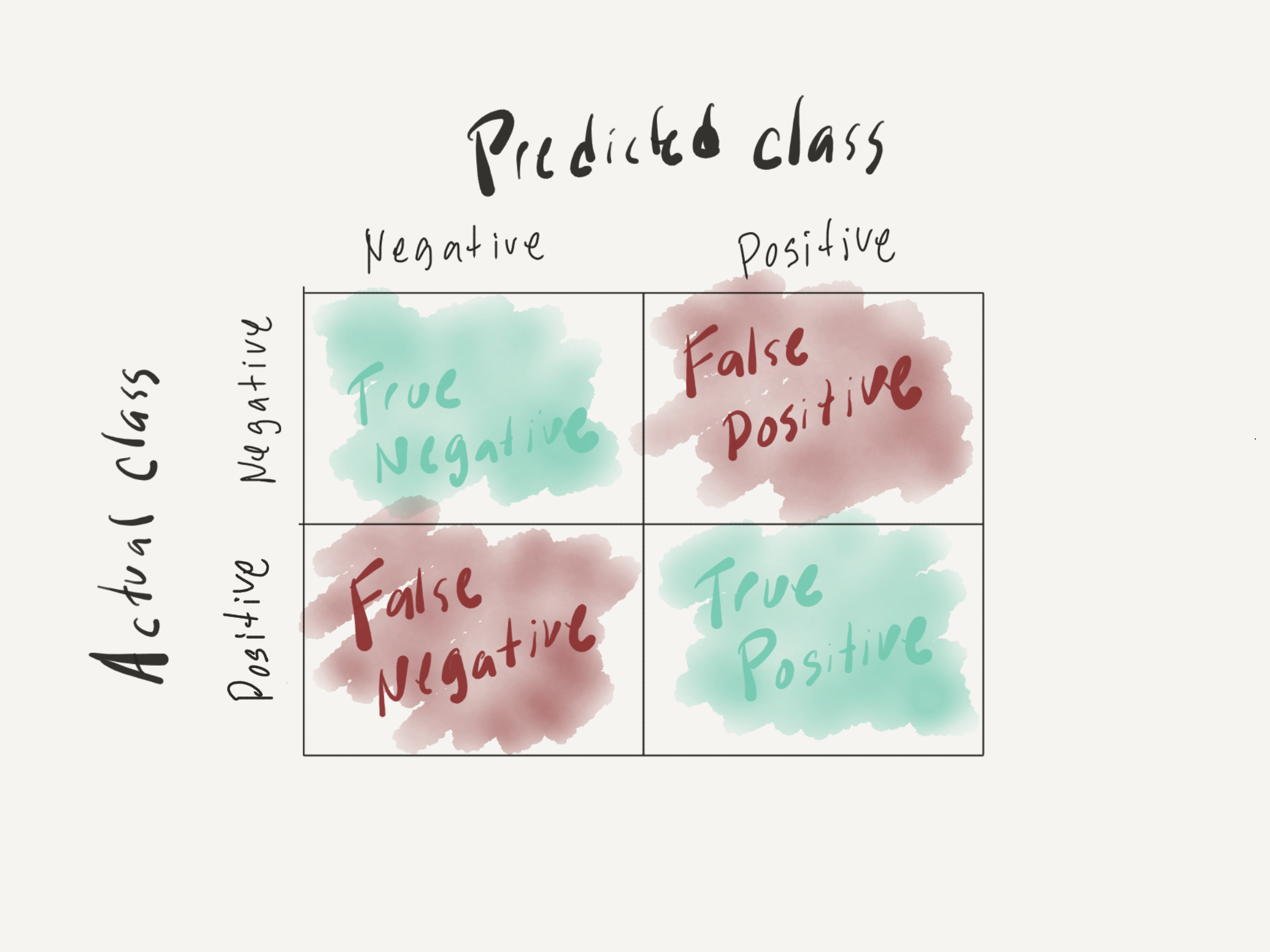
Figure 9.1: Confusion matrix